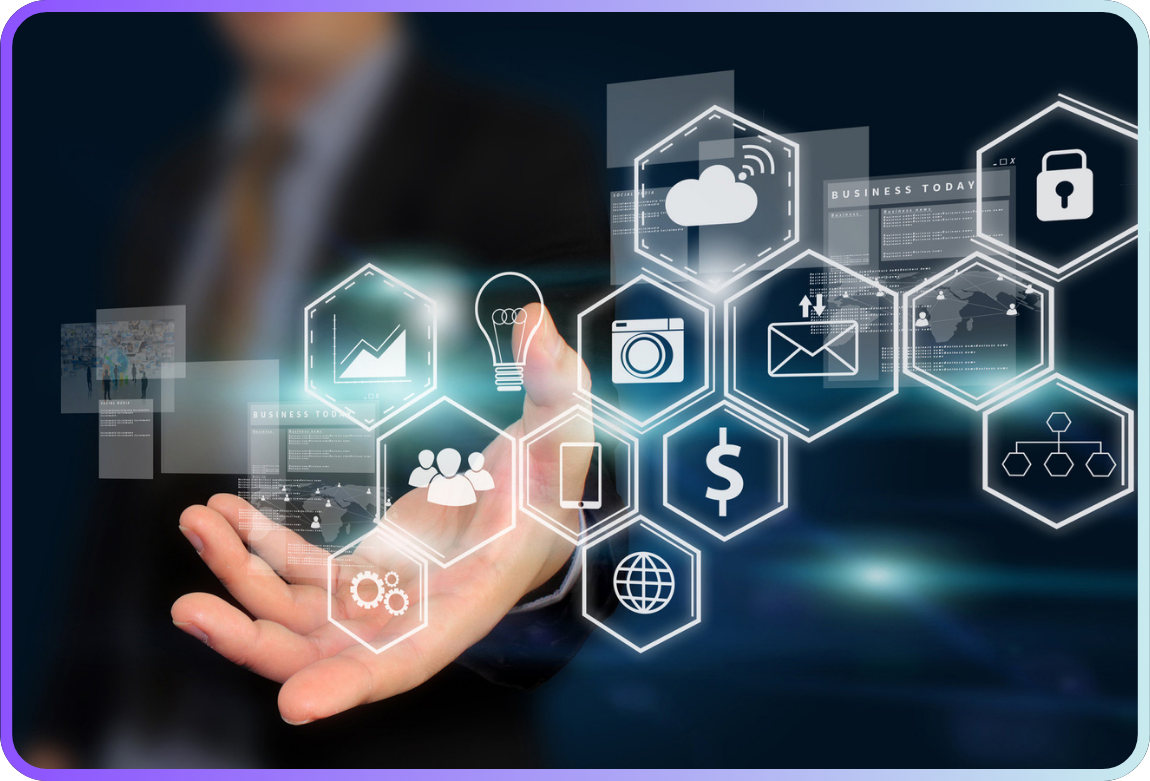
In the age of digital transformation, the most critical competitive advantage for financial institutions lies in their ability to make sense of data and take rapid action. At this point, AI technologies in banking—especially when integrated with analytic banking applications—are reshaping the rules of the game in the sector.
Artificial intelligence not only analyzes data but also offers recommendations, makes predictions, and provides systems capable of making human-like decisions. In this context, Generative AI is ushering in a new era in customer experience and decision support systems with its ability to generate content and scenarios from data.
Below, we explore how AI technologies function across different areas of the banking sector, including their technical aspects:
1. Generative AI and Natural Language Processing (NLP) in Customer Interaction
Modern customer service is being transformed by generative AI-powered virtual assistants (e.g., ChatGPT, Bard). These systems go beyond responding to simple commands and can:
• Conduct sentiment analysis to understand the customer’s emotional state,
• Offer personalized suggestions based on transaction history,
• Automatically generate training materials from customer feedback.
Bank applications now commonly feature AI agents that converse naturally and provide user-specific suggestions. These systems typically consist of two core components integrated into CRM infrastructures:
• NLP models analyze customer messages to detect intent and sentiment, enabling automatic routing without the need for a human agent.
• Generative AI models produce original, contextual responses and can even generate content (e.g., campaign messages or summaries).
Together, these technologies enable real-time and personalized customer experiences.
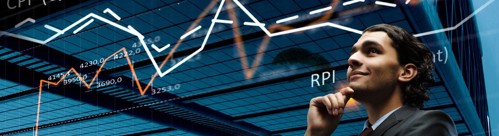
2. AI-Powered Predictive Models in Credit Scoring
Traditional credit scoring models mostly rely on regression techniques and a limited number of variables. In contrast, AI-enhanced models utilize high-dimensional datasets, including:
• New-generation features such as payment behavior, digital activity, device data, and transaction speed,
• Time-series analysis to predict late payment risks,
• Supervised learning algorithms like XGBoost, LightGBM, and Random Forest, which increase accuracy in credit assessments.
As a result, banks can issue loans with reduced default risk across both individual and commercial segments.
3. Fraud Detection through Anomaly Detection and Graph-Based Models
Fraud detection is evolving from rule-based systems to anomaly detection approaches. These systems:
• Use unsupervised or supervised learning to identify abnormal behavior based on historical patterns. While unsupervised methods detect anomalies in unlabeled data, supervised models learn from known fraud cases for more precise classification.
• Apply Graph Neural Networks (GNNs) to analyze customer networks and detect related fraudulent accounts.
• Utilize real-time monitoring with features like transaction speed fluctuations and IP/behavior inconsistencies.
With online learning, these models can continuously adapt to new fraud techniques.
4. Personalized Product and Campaign Recommendations via AI-Based Segmentation
AI analyzes customer data to create highly accurate micro-segments using:
• Clustering algorithms like K-means, DBSCAN, and Gaussian Mixture Models based on spending and product usage behavior,
• Association rule learning (e.g., Apriori, FP-growth) to identify cross-sell opportunities,
• Generative AI to automatically generate tailored offer texts and campaign suggestions for each segment.
This structure helps banks increase revenue per customer and optimize marketing budgets.
5. Analytic Banking: Decision Support Systems and Reporting
Analytic Banking refers to AI-powered strategic decision-making systems in banks. In this domain:
• Predictive analytics forecasts credit demands, liquidity needs, and product preferences.
• Prescriptive analytics offers scenario analysis and optimization by answering “what should be done?”.
• Generative AI can produce strategic reports, executive summaries, and headline recommendations for decision-makers.
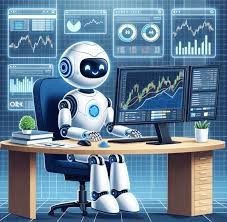
For instance, a GPT-powered model could present a CEO with visualized profitability data and narrative insights for a regional loan product.
6. Portfolio Management and Robo-Advisory Systems
In the investment field, AI provides:
• Mean-variance optimization combined with AI to generate dynamic, risk-adjusted portfolio recommendations,
• Sentiment analysis to gauge market mood from social media and news sources,
• Generative AI to produce personalized reports, alerts, and investment insights.
These systems offer retail investors access to professional-grade advisory services.
7. Regulatory Compliance (RegTech) and ESG Analytics
Complying with financial regulations can be both costly and complex. AI helps by:
• Using NLP to analyze regulation documents and extract actionable insights,
• Automatically generating compliance reports,
• Analyzing Environmental, Social, and Governance (ESG) data for sustainability reporting.
Generative AI accelerates this process with functions like text summarization, report generation, and data interpretation.
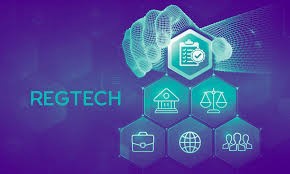
Conclusion: Intelligent and Analytical Banking Through AI
AI and banking are now inseparable. Thanks to AI, banks are no longer just institutions that process transactions—they’re becoming learning, recommending, and predictive ecosystems.
With analytic banking frameworks and generative AI solutions, all processes—from customer interaction to strategic decision-making—are becoming smarter. Banks that move beyond just storing data to interpreting and acting on it will gain a competitive edge.
However, AI models aren’t always guaranteed to be fair or reliable. Models trained on biased data can reflect that bias in their decisions. Furthermore, malicious data manipulation can lead models to produce flawed outcomes. Techniques like adversarial training can be employed to improve the robustness and security of these systems.
In short, AI continues to be the driving force of technological transformation in banking. For banks today, AI is not an option but a necessity for sustainable growth.
References:
- Banking 4.0: Artificial Intelligence (AI) in Banking Industry & Consumer’s Perspective
- Artificial Intelligence (AI) in the Banking Industry: A Review of Service Areas and Customer Service Journeys in Emerging Economies | Business & Management Compass
- Figure1: https://on.com.tr/sozluk/bankacilik-ve-finansta-yapay-zeka-kullanimi#:~:text=Bankacılık%20ve%20finansta%20yapay%20zekanın,daha%20hızlı%20geri%20dönüşler%20sağlar.
- Figure2: The use of Artificial Intelligence in the Banking Industry | IEEE Conference Publication | IEEE Xplore
- Figure3: Bankacılık Sektöründe Yapay Zeka: Geleceğin Finansal Dönüşüm | Nurol Portföy