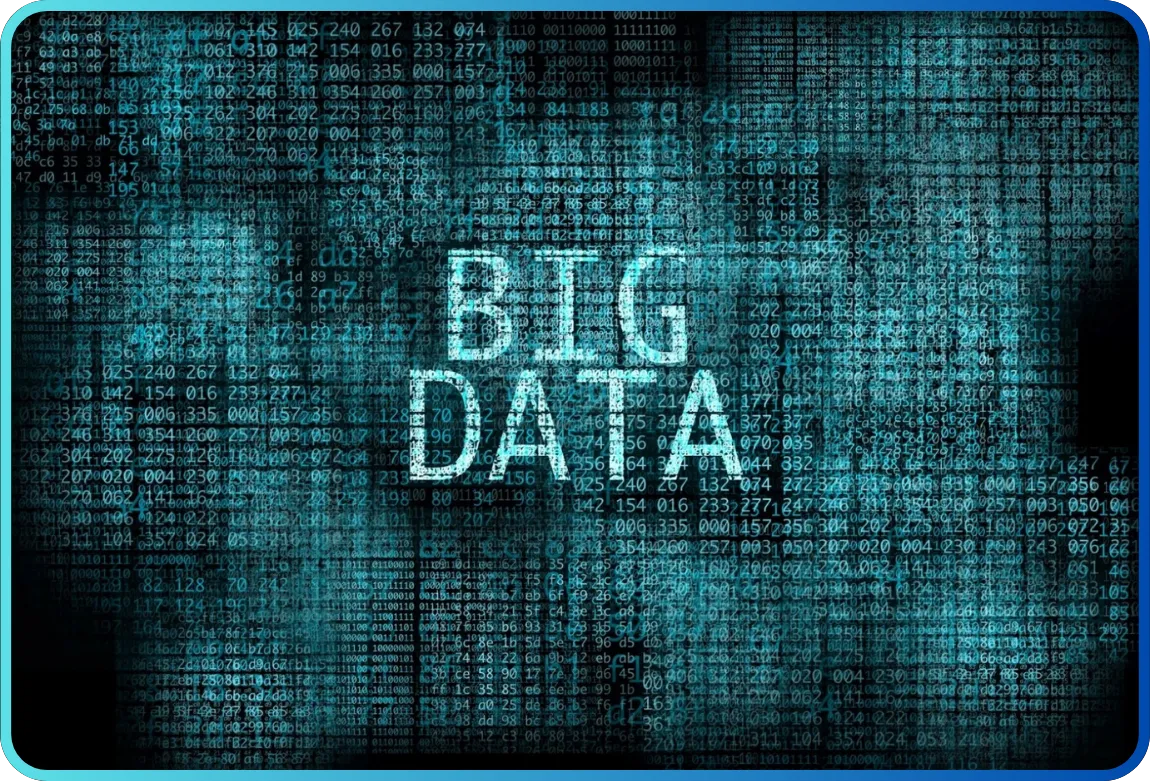
As companies continue to develop data-driven strategies, making sense of big data is becoming critical competency for gaining competitive advantage. In this article, we will explore the increasingly popular concept of big data – its sources, challenges, application areas, and its role in the financial sector – supported by examples based on customer analytics.
What is “big data”?
The term of “big data” refers to data sets that are too large, fast, or complex to processed using traditional database methods. There characteristics are commonly referred to as the “3V’s of Big Data”: Volume, Velocity, Variety (i). As shown in the figure below, depending on specific needs, two additional dimensions are often added to this concept: Veracity and Value. (Figure 1)
Figure 1. 5V's of Big Data (ii)
Let's give examples of the sources of big data: social media platforms that generate vast amounts of data in real-time from millions of users, smartwatches that constantly collect data, GPS systems, traffic cameras, sensors, IoT devices, banking transactions, and financial data produced through credit card usage (iii). As there are many more examples, it becomes increasingly clear how naturally and pervasively big data is being produced within our daily routine. While individuals unconsciously contribute to this data generation every day, companies are striving to process and extract value from this massive resource. The key to understanding customers – an indispensable priority for organizations – lies in analyzing this data and integrating it into decision-making system. Indeed, we can observe that the business world is increasingly adopting “data-driven” approaches as a core mindset.
What is done with big data?
By analyzing the continuous flow of big data in real-time, faster, and more effective decision-making mechanisms are being established. For instance, Amazon can analyze customer behavior instantly to apply dynamic pricing strategies (iv). Similarly, companies can uncover user preferences through big data, enabling personalized product recommendations and targeted campaigns to create sales opportunities and increase customer engagement. The playlists and suggested movies we see on popular entertainment and music apps are not coincidences; they are outcomes of machine learning and artificial intelligence applications powered by big data. Additionally, big data generated by users is processed through AI models to enhance the safety of autonomous driving and provide more accurate road and traffic information.
So, what is the finance and banking sector doing with big data?
The finance and banking sector, to which we contribute with our expertise, has started to adopt a “data-driven banking” approach, recognizing the value of the resources it has. Through this approach, analytical models developed using big data sources aim to better understand customers, improve decision-making processes, and enhance operational efficiency. Within the banking domain, the use of big data is crucial for offering the right product at the right time, ensuring seamless customer experiences, managing risk effectively, and preventing fraudulent activities.
Big data clusters generated from account and credit transactions, card expenditures, and mobile or online banking usage are used for developing various customer analyses and AI models. For example, through advanced customer segmentation, banks can identify high-income individuals, investors, credit-seekers, or customers facing payment difficulties, and offer them personalized services such as special pricing, financial advisory, or credit and card offers. J.P Morgan, with a customer base exceeding 3 billion, utilized big data that include customer spending behavior to develop personalized marketing strategies. One highlighted application is offering different campaigns to customers who shop physically versus online (v).
Additionally, customer churn prediction models are also designed to anticipate attrition and deliver personalized offers, products or benefits to retain customer. Algo-trading systems capable of execute transactions within seconds in financial markets, and robo-advisory systems recommending investment portfolio tailored to client profiles, are being developed. While the main goal of these applications is to enhance the customer experience, big data-powered customer analytics also generate valuable outcomes for a vital aspect of sustainability: security.
Cybersecurity systems and fraud detection tools operate on big data. While banks strive to secure their own data, they also use real-time abnormal transaction detection system powered by big data to protect customer accounts and prevent credit card fraud. Although security is the primary goal, these systems rely on advanced behavior-based models built on customer account activities, transactional data, locational data, and device information – ultimately producing critical results that both add value to the institution and benefit the customer. For instance, the case of Danske Bank, one of the Denmark’s largest banks, has clearly demonstrated the impact of big data in fraud detection. Previously, the bank’s traditional system had only a 40% success rate and generated around 1200 false positives per day. By partnering with analytics provider to develop models using big data analytics and machine learning, they improved fraud detection rate by 50% and reduced false positives by 60%. As a result, both customer satisfaction and the bank’s operational efficiency increased significantly (vi).
But how is customer analytics performed with big data?
While big data offers tremendous opportunities and valuable outcomes, as discussed as so far, it also brings significant challenges. When working with big data, the fundamental steps of data mining – such as data collection, cleaning, processing, and modelling – are still followed. However, what sets the process apart is the technology stack used. (Figure 2)
Due to its nature, big data consists of massive and structured or unstructured datasets, making traditional data processing methods insufficient for handling data at this scale. Storing millions of continuously growing records in an efficient and organized way using conventional systems would lead to high costs. Therefore, cloud storage solutions like Amazon S3 and Google Cloud, as well as distributed file systems like Hadoop, are commonly utilized. Once data collection and storage are handled, data quality issues often arise. Since the data comes from various sources and formats, cleaning, standardizing, and integrating the data becomes a challenge. At this stage, using proposer ETL pipelines and tools such as Apache NiFi significantly accelerates the integration process.
To handle continues data flow and perform real-time analytics, low latency and high computational power are essential. Tools like Apache Kafka, Apache Flink, and Spark Streaming enable real-time processing of large-scale data streams. As the datasets grows, model training and prediction times increase, potentially affecting model performance. Achieving higher accuracy may also require longer processing times. At this point, not only is the selection of right AI models crucial, but also the use of scalable infrastructures like Apache Spark with distributed analytics capabilities and high processing power becomes essential.
Beyond technical challenges, there is also a growing need for qualified professionals. It becomes inevitable to require skilled big data analysts who are proficient in the technologies mentioned. All these factors make working with big data a comprehensive challenge that must be addressed from multiple perspectives – including data management, analytics, infrastructure, and skilled human resources.
Figure 2. Top Big Data Technologies & Techniques (vii)
For companies, working with big data involves more than just collecting information; it requires a strategic plan built on a robust technological infrastructure. For any company aiming to benefit from advantages of big data, focusing on this strategy will be a vital step towards providing a better experience to its customers.
Resources: